Introduction
Aortic aneurysm (AA) is a localized, progressive degenerative processes of the aortic wall that leads to fixed dilatation to a diameter exceeding 1.5-fold the normal adjacent segment or vessel diameter; AA most commonly occurs in the infrarenal aorta [1]. Thoracic aortic aneurysm (TAA) and abdominal aortic aneurysm (AAA) are the most common types of AA encountered in clinical practice [2]. Although they have similar physical characteristics, their pathophysiological changes are not exactly identical, and AAA has a higher incidence rate [3, 4]. Previous epidemiological investigations have shown that the prevalence of AAA in older men varies, ranging from 6.2% in Italy to 1.7% in England [5]. In the United States, AAA alone has become one of the top 20 causes of death and a serious medical burden [6]. Although extensive research has examined the risk factors for AA, such as hypertension, advanced age, and sex, the gut microbiota is a new research direction.
The human gut microbiota is a complex system that may influence the progression of diseases, such as atherosclerosis, by regulating metabolic and immune modulation pathways [7]. Changes in gut microbial diversity have also been observed in populations with diabetes and hypertension [8, 9]. Recent studies have suggested that the gut microbiota may influence AA occurrence and progression. For example, gut microbiota metabolites can lead to aneurysm formation through mechanisms involving inflammation and apoptosis [10]. R. intestinalis and its metabolites affect the progression of AAA by influencing the formation of neutrophil extracellular traps [11]. Additionally, alterations in the gut microbiota have been found to affect the occurrence or progression of intracranial aneurysms [12, 13] or AAA [14]. These findings suggest that a specific gut microbiota may play major roles in the occurrence and development of AA and its subtypes. However, owing to various biases in traditional epidemiological studies and confusion due to reverse causality, the causal relationship between a particular gut microbiota and AA remains unclear.
Mendelian randomization (MR) is a statistical method based on whole-genome sequencing data used to uncover causal relationships. On the basis of the principles of MR, different genotypes determine different intermediate phenotypes. If these phenotypes represent specific exposure characteristics of individuals, then the association effects between genotypes and diseases can represent the exposure effect of the factor on the disease. Because allelic genes follow the principles of random allocation, this effect is not influenced by the confounding factors and reverse causal associations commonly found in traditional epidemiological studies. Therefore, MR provides a method to explore causal relationships between exposure and outcomes while minimizing the influence of confounding factors to the greatest extent [15]. The objective of this study was to investigate the causal relationship between the genetically predicted gut microbial composition and AA, including the subtypes TAA and AAA, through a two-sample MR approach. Our findings may provide guidance for the development of useful microbial biomarkers as potential therapeutic targets for AA and its subtypes.
Methods
Figure 1 illustrates the analytical flow of this study.
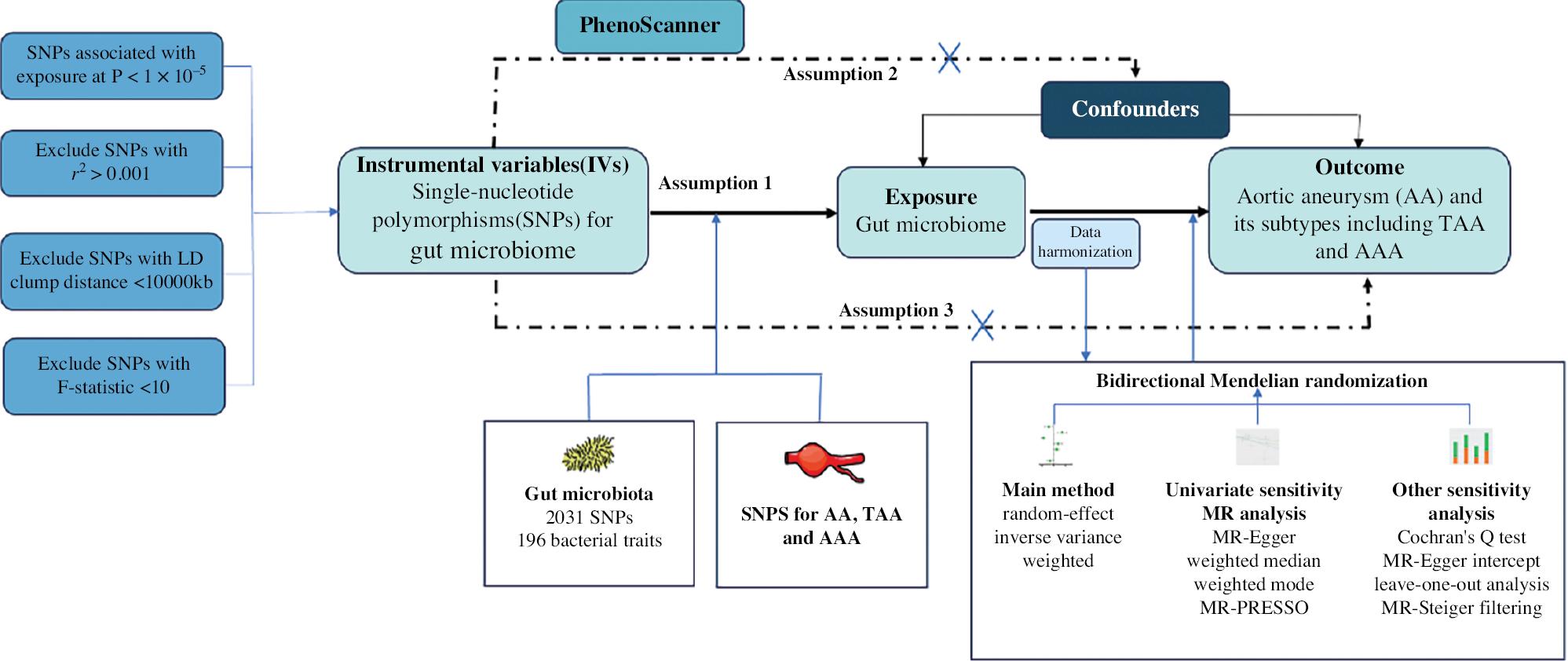
Study Design for MR Analysis of the Associations Between the Gut Microbiota and Aortic Aneurysm.
MR, Mendelian randomization; AA, aortic aneurysm; TAA, thoracic aortic aneurysm; AAA, abdominal aortic aneurysm.
Data Source for Gut Microbiota
The comprehensive genome-wide association study (GWAS) data regarding the composition of the human gut microbiome came from a large-scale multi-ethnic GWAS meta-analysis. The MiBioGen study, the largest and most diverse research aimed at studying host-genetics-microbiome associations, involves 18,473 individuals from 24 European, East Asian, American, and other cohorts. Multiple hypervariable regions (i.e., V1-V2, V3-V4, and V4) in the 16S ribosomal RNA (rRNA) gene were used to investigate the composition of the gut microbiota. A total of 122,110 single nucleotide polymorphisms (SNPs) in the 16S fecal microbiome were analyzed in 18,473 individuals [16, 17]. After exclusion of 15 microbial taxa without specific species names, a total of 196 bacterial groups of the 211 total bacterial taxa were included.
Data Source for Outcomes
The GWAS summary statistics for AA were obtained from FinnGen version 9 (https://www.finngen.fi/en), a medical project aimed at analyzing genomic and health data for participants in the Finnish biobank to identify genotype-phenotype correlations. The model included factors such as sex, age, and ten principal components as covariates [18]. The analysis process is detailed in the following link: (https://finngen.gitbook.io/documentation/methods/phewas). The study population includes 349,539 controls, 7395 patients with AA, 3548 patients with AAA, and 3510 patients with TAA. AA included ruptured thoracic aortic aneurysms; thoracic aortic aneurysms without mention of rupture; ruptured abdominal aortic aneurysms; abdominal aortic aneurysms without mention of rupture; ruptured thoracoabdominal aortic aneurysms; thoracoabdominal aortic aneurysms without mention of rupture; ruptured aortic aneurysms at unspecified sites; and aortic aneurysms at unspecified sites, without mention of rupture. AAA included ruptured abdominal aortic aneurysms and abdominal aortic aneurysms without mention of rupture. TAA included ruptured thoracoabdominal aortic aneurysms and thoracoabdominal aortic aneurysms without mention of rupture. The diagnosis of AA and its subtypes was made with nationwide registries harmonized over International Classification of Diseases (ICD) revisions 8, 9, and 10; cancer-specific ICD-O-3; and NOMESCO procedure codes (https://www.finngen.fi/en/researchers/clinical-endpoints).
Selection of Instrumental Variables
To ensure adequate selection of instrumental variables (IVs), we chose SNPs with P-values below the genome-wide significance threshold (1 × 10−5). Furthermore, we evaluated and excluded IVs with an F-statistic (formula: , where N represents the sample size) <10, to ensure the strength of the association between IVs and the exposure [19]. Additionally, to ensure the independence of SNPs, we set the linkage disequilibrium parameter (R2) of SNPs to 0.001 and considered a genetic distance of 10,000 kb. To prevent potential pleiotropy, we used PhenoScanner [20] to examine potential confounders associated with IVs, such as smoking and atherosclerosis, to mitigate their influence on the relationship between the exposure and outcomes (Supplementary Table 5). If the number of SNPs in the IVs selected by a certain microbial community was less than 2, the microbial community data were not included in the final results, because methods such as MR-Egger and leave-one-out could not be used for validation.
Statistical Analysis
Before conducting MR analysis, we first harmonized the IV SNPs. In this two-sample MR analysis, to investigate the causal relationship between the exposure factor and the outcome, we used the inverse variance weighted (IVW), MR-Egger, weighted median, and weighted mode methods. IVW is a classical MR analysis method that combines Wald ratio estimates of each IV into a meta-analysis. The MR-Egger method is based on the assumption of the Instrument Strength Independent of Direct Effect (InSIDE) and considers a certain degree of horizontal pleiotropy in the statistical process [21]. The weighted median allows for the inclusion of certain genetic variants that may be invalid [22]. The weighted mode enables certain IVs to not meet the requirements of MR inference when most IVs have similar causal estimates. Among these methods, the random-effects IVW method is the primary analysis method, which is used to perform a meta-analysis of SNP-specific Wald ratios, on the basis of an assumption of balanced horizontal pleiotropy [23]. If the above results are inconsistent, priority should be given to the IVW results as the primary result. The design of this study followed the STROBE-MR guidelines [24].
Sensitivity Analysis
Several statistical tests were used to examine the presence of violations of the assumptions of MR, particularly horizontal pleiotropy. First, Cochran’s Q statistic was calculated to quantify heterogeneity in the effects generated by IVs. Second, the MR-Egger intercept was assessed for deviation from zero to detect horizontal pleiotropy [19]. MR Pleiotropy Residual Sum and Outlier (MR-PRESSO) tests were applied to test for horizontal pleiotropy and outliers. Compared with MR-Egger, MR-PRESSO has greater accuracy in identifying horizontal pleiotropy and outliers [25]. In cases in which the MR-Egger and MR-PRESSO results were inconsistent, priority was given to the MR-PRESSO results as the main findings. Additionally, leave-one-out analysis was conducted to detect any heterogeneity driven by individual SNPs. The MR-Steiger test was used to evaluate and filter out SNPs that might indicate reverse causality relationships [26]. Furthermore, reverse causality analysis was performed to examine reverse causality relationships. To account for multiple testing, we applied Bonferroni correction with significance thresholds set at for each category level, for example, class
. Statistical analysis was performed in R software version 4.2.3 (https://www.r-project.org/).
Results
Selection of Instrumental Variables for Gut Microbiomes
SNPs were selected as IVs within the gene locus range (P < 1 × 10−5). After removal of SNPs with low linkage disequilibrium effects and weak associations with the exposure factor (F < 10), a total of 2031 SNPs were retained and included in the analysis. These SNPs included 102 SNPs from 9 phyla, 179 SNPs from 16 classes, 217 SNPs from 20 orders, 341 SNPs from 31 families, and 1192 SNPs from 119 genus. The main information collected for these SNPs included the effect allele, other allele, standard error, P value, and other relevant data for analysis purposes.
Causal Effects of the Gut Microbiota on AA and its Subtypes
Two-sample MR analysis was used to identify causal relationships between the gut microbiota and aneurysms. Higher genetic predictions of the genus Family XIII UCG001 and genus Parasutterella were associated with greater risk of AA, whereas higher genetic predictions of the class Actinobacteria, class Lentisphaeria, family Bifidobacteriaceae, genus Blautia, order Bifidobacteriales, order Victivallales, and phylum Lentisphaerae were associated with lower risk. For the TAA subtype, on the basis of the IVW results, 13 gut microbial taxa were determined to have a causal relationship with TAA risk. Among them, the class Actinobacteria, family Bifidobacteriaceae, family Clostridiales vadin BB60 group, genus Coprococcus1, genus Veillonella, genus Intestinibacter, order Bifidobacteriales, order Victivallales, and phylum Lentisphaerae were associated with a lower TAA risk, whereas the family Oxalobacteraceae, family Pasteurellaceae, genus Eubacterium xylanophilum group, and genus Ruminococcaceae UCG014 were associated with higher TAA risk. Thirteen gut microbial taxa associated with AAA risk were also identified in the control and case groups (Supplementary Table 1). Among them, the class Lentisphaeria id.2250, family Victivallaceae id.2255, genus Lactococcus id.1851, genus Anaerotruncus id.2054, genus Blautia id.1992, and order Victivallales id.2254 were associated with lower AAA risk, whereas the genus Catenibacterium id.2153, genus Family XIII AD3011 group id.11293, genus Family XIII UCG001 id.11294, genus Oxalobacter id.2978, genus Bilophila id.3170, genus Escherichia Shigella id.3504, and genus Eubacterium brachy group id.11296 were associated with higher AAA risk. The relationship between the genus Family XIII AD3011 and AAA showed evidence of pleiotropy according to the MR-Egger method but no pleiotropy according to the MR-PRESSO method. On the basis of the overall results, the MR-PRESSO test was deemed reliable, indicating no horizontal pleiotropy. The above results are shown in Figures 2–4. Cochran’s Q test did not reveal significant heterogeneity (P > 0.05), and the MR-Egger and MR-PRESSO tests did not identify significant horizontal pleiotropy or outliers (P > 0.05) (Supplementary Table 1). The MR Steiger test showed no evidence of reverse causality (Supplementary Table 2).
Bonferroni-Corrected Test
After Bonferroni correction, the results (Table 1) showed strong causal relationships between certain microbial taxa and aneurysms.
MR Analysis Results of the Causal Relationship between the gut microbiota and AA.
Outcome | Classification | Nsnp | Methods | OR (95% CI) | P Value |
---|---|---|---|---|---|
AA | Phylum Lentisphaerae | 9 | Inverse variance weighted | 0.82 (0.73–0.92) | 0.001081677 |
MR Egger | 0.73 (0.45–1.18) | 0.240365964 | |||
Weighted median | 0.85 (0.73–0.98) | 0.030212552 | |||
Weighted mode | 0.88 (0.72–1.09) | 0.275066916 | |||
Class Lentisphaeria | 8 | Inverse variance weighted | 0.81 (0.71–0.93) | 0.002812266 | |
MR Egger | 0.73 (0.44–1.22) | 0.272388475 | |||
Weighted median | 0.83 (0.69–0.99) | 0.035462053 | |||
Weighted mode | 0.89 (0.71–1.12) | 0.352134357 | |||
Family Bifidobacte\riaceae | 11 | Inverse variance weighted | 0.79 (0.70–0.89) | 8.00528 × 10−5 | |
MR Egger | 0.93 (0.55–1.57) | 0.787451213 | |||
Weighted median | 0.79 (0.63–0.98) | 0.034571324 | |||
Weighted mode | 0.78 (0.59–1.04) | 0.118112729 | |||
Genus Family XIII UCG001 | 8 | Inverse variance weighted | 1.33 (1.14–1.55) | 0.000203273 | |
MR Egger | 1.10 (0.61–2.00) | 0.765969749 | |||
Weighted median | 1.46 (1.12–1.91) | 0.005139698 | |||
Weighted mode | 1.47 (0.99–2.20) | 0.10001866 | |||
TAA | Phylum Lentisphaerae | 9 | Inverse variance weighted | 0.81 (0.70–0.93) | 0.002494393 |
MR Egger | 0.65 (0.35–1.21) | 0.217125199 | |||
Weighted median | 0.82 (0.65–1.03) | 0.084715554 | |||
Weighted mode | 0.96 (0.68–1.35) | 0.808702009 | |||
Family Bifidobacteriaceae | 11 | Inverse variance weighted | 0.71 (0.61–0.82) | 1.91475 × 10−6 | |
MR Egger | 0.94 (0.44–1.99) | 0.870227065 | |||
Weighted median | 0.70 (0.51–0.96) | 0.025209257 | |||
Weighted mode | 0.68 (0.46–1.01) | 0.082613371 | |||
Genus Ruminococcaceae UCG014 | 10 | Inverse variance weighted | 1.30 (1.13–1.49) | 0.000255698 | |
MR Egger | 1.14 (0.66–1.98) | 0.645934749 | |||
Weighted median | 1.17 (0.85–1.61) | 0.324232843 | |||
Weighted mode | 1.14 (0.78–1.66) | 0.52566428 | |||
AAA | Genus Bilophila | 13 | Inverse variance weighted | 1.36 (1.17–1.57) | 0.0000452 |
MR Egger | 1.55 (0.48–5.03) | 0.483243703 | |||
Weighted median | 1.34 (0.98–1.85) | 0.068775942 | |||
Weighted mode | 1.29 (0.80–2.08) | 0.309338759 |
In AA, lower levels of the class Lentisphaeria (0.81, 95% CI: 0.71–0.93, P < 0.003), family Bifidobacteriaceae (0.79, 95% CI: 0.70–0.89, P < 0.0016), and phylum Lentisphaerae (0.82, 95% CI: 0.73–0.92, P < 0.005), as well as higher levels of the genus Family XIII UCG001 (1.33, 95% CI: 1.14–1.55, P < 0.0016), were strongly associated with AA. For the genus Blautia, because the IV contained only two SNPs, further sensitivity analysis could not be conducted; therefore, this genus was not included in the final results. Using leave-one-out analysis to sequentially remove SNPs, the predicted results for the remaining SNPs remain stable, thus indicating that the causal relationship was not driven by specific SNPs (Supplementary Figures 1–4).
In TAA, lower levels of the family Bifidobacteriaceae id.433 (0.71, 95% CI: 0.61–0.82, P < 0.0016) and phylum Lentisphaerae id.2238 (0.81, 95% CI: 0.70–0.93, P < 0.005), and higher levels of the genus Ruminococcaceae UCG014 id.11371 (1.30, 95% CI: 1.13–1.49, P < 0.0004), were strongly associated with TAA. Leave-one-out analysis did not show significant changes in the risk estimations (Supplementary Figures 5–7).
In AAA, higher levels of the genus Bilophila id.3170 (1.36, 95% CI: 1.17–1.57, P < 0.0004) were closely associated with AAA incidence. All SNP-specific F-statistics were greater than 10 (Supplementary Table 3), and MR Steiger tests (Supplementary Table 2) indicated no evidence of reverse causation. The forest plot results are shown in Supplementary Figure 8. The leave-one-out analysis also yielded stable results after iterative exclusion of each SNP (Supplementary Figure 8).
Reverse Analysis
The reverse causation analysis results (Supplementary Table 4) indicated that only AA might lead to higher levels of the genus Subdoligranulum (OR = 1.07, 95% CI: 1.03–1.11, P < 0.001) whereas the other results did not show statistical significance (P > 0.05).
Discussion
Previous studies on the relationship between AA and the gut microbiota were conducted primarily in animal models or prospective clinical trials [10, 11, 27]. However, prospective randomized trials are time-consuming and costly, and confounding factors are difficult to control. In such cases, genotypes can be considered to replace exposure factors for causal inference, in a process akin to a natural randomized controlled trial. A recent study has explored the relationship between AAA and the gut microbiota through MR and arrived at conclusions similar to ours. Therefore, we further extended our investigation to explore the relationship between AA and its subtypes, including TAA and AAA, with the gut microbiota. Additionally, we conducted Bonferroni correction to enhance the credibility of the results [28]. In this study, we used MR to analyze the potential causal relationship between AA and its subtypes and the gut microbiota at the genetic prediction level. Our results were based on a large GWAS database, to achieve reliable causal interpretation effects. Our findings may provide guidance for future AA treatment targeting specific gut bacteria in a genomic context.
We identified 26 microbial communities associated with the incidence of AA and its subtypes, six of which showed strong causal relationships. Among them, the genetic prediction level of the phylum Lentisphaerae may be associated with diminished risk of AA and TAA. In contrast, the class Lentisphaeria, a member of the phylum Lentisphaerae, was associated with a lower incidence of only AA but was associated with a lower risk of AAA before Bonferroni correction. The phylum Lentisphaerae, a member of the Planctomycetes-Verrucomicrobia-Chlamydia superphylum, is currently divided into two classes: Lentisphaeria and Oligosphaeria [29, 30]. Lentisphaeria includes two orders (Lentisphaerales and Victivallales) and two families (Lentisphaeraceae and Victivallaceae). Previous studies have suggested that Lentisphaerae bacteria promote polysaccharide degradation, facilitate phosphorus and iron metabolism, and increase and maintain microbial diversity [31]. Studies have found that Lentisphaerae dysbiosis is associated with ileitis [32], autoimmune hepatitis [33], and systemic sclerosis [34], through mechanisms potentially associated with inflammation, whereas the inflammatory response may promote the progression of AA through pathways such as atherosclerosis. However, because Lentisphaerae members are difficult to culture, the above conclusions are based on the results of metagenomic predictions, and further experimental testing or clinical validation is necessary in the future to confirm the cellular biology functions of Lentisphaerae bacteria and their role in the occurrence of AA.
Herein, higher levels of the gene-predicted family Bifidobacteriaceae were associated with lower risks of AA and TAA. Bifidobacterium is an anaerobic, bile-resistant, Gram-negative rod-shaped bacterium [35]. Studies have shown that Bifidobacterium may directly regulate intestinal function by modulating the expression of genes associated with nutrient absorption, mucosal barrier enhancement, and vascular endothelial growth factor expression [36]. AA is relatively more common in older people [37], whose gut microbiota is characterized by diminished Bacteroides spp., Bifidobacterium spp., and Lactobacilli spp. Chiara et al. [38] have found that older people who consume probiotics have elevated levels of the family Bifidobacteriaceae in their gut microbiota, along with elevated total short-chain fatty acids (SCFAs) and butyric acid levels in fecal samples, and diminished serum hsCRP. SCFAs include primarily acetate, butyrate, and propionate, which are metabolites of the intestinal microbiota, and have functions in regulating the immune system and maintaining the intestinal epithelial barrier [39]. Therefore, the family Bifidobacteriaceae may suppress the occurrence of AA and TAA by maintaining intestinal function and exerting anti-inflammatory effects.
The genera Blautia and Ruminococcaceae UCG014 both belong to the phylum Bacillota. Interestingly, although further sensitivity analysis could not be conducted, our results nonetheless suggested that the gene-predicted level of the genus Blautia negatively correlated with the occurrence of AA and AAA, whereas that of the genus Ruminococcaceae UCG014 positively correlated with TAA occurrence. Blautia is a symbiotic strict anaerobe that has been reported to prevent inflammation and maintain intestinal balance environment through the production of SCFAs and upregulation of regulatory T cells in the gut [40]. The abundance of Blautia is diminished in patients with inflammatory bowel disease [41] and colorectal cancer [42]. Thus, Blautia is a probiotic with positive effects on human health [43]. The genus Ruminococcaceae UCG014 also has a role in producing SCFAs [44]; however, our study suggested that it is associated with a high risk of TAA; further research may be necessary to confirm this finding.
In addition, our results indicated that the genus Bilophila and genus Family XIII UCG001 were significantly associated with elevated risk of AAA and AA, respectively. Bilophila species have been shown to promote intestinal barrier dysfunction, bile acid metabolism disorders, and inflammation in mouse models [45]. In vitro experiments have shown that Bilophila species can convert taurine to hydrogen sulfide, which may play an important role in systemic inflammation [46]. However, the relationship between genus Family XIII UCG001 and diseases has not been clearly demonstrated. In an experiment conducted using mice as experimental subjects, the results suggested that the abundance of genus Family XIII UCG001 may be associated with arachidonic acid [47], and arachidonic acid and its metabolites have been associated with various cardiovascular diseases [48]. Both these genera may affect the development of AA or AAA through inflammatory responses.
Our study has several limitations. First, the gut microbiota exhibits high heterogeneity and interindividual variability, thus significantly decreasing the statistical power of microbiome GWAS analysis and potentially leading to pleiotropy. Therefore, we counted only IVs with F statistics greater than 10 and used various sensitivity analyses to decrease the effects of weak instrument bias and multicollinearity variability. Second, MR analysis is based on untestable assumptions, and further experimental and clinical validation studies are required to assess the clinical significance of microbial species. Furthermore, because of the lack of population demographic data, we were unable to perform subgroup analyses, such as investigation of the causal relationship between sex- and age-specific gut microbiota and AA. Finally, as described earlier, variations in the incidence of AAA occur among countries, and populations in different geographical regions also exhibit distinct gut microbiota characteristics [49]. Subsequent validation with GWAS data from more countries is necessary.
Conclusion
The occurrence of AA and its subtypes is closely associated with the gut microbiota. Reverse causal analysis did not indicate a significant influence in the presence of gut microbiota that promote or inhibit AA and its subtypes. Interestingly, the gut microbiota affecting the occurrence of AAA or TAA do not overlap, thus potentially further indicating that AAA and TAA have different pathogenic mechanisms. We may further investigate these specific microbial communities to explore their roles in AA and its subtypes. Our findings may potentially provide guidance for the development of potential therapeutic targets for AA and its subtypes from the perspective of the gut microbiota. In summary, we used gene prediction to determine specific gut microbiota that might serve as useful biomarkers or therapeutic targets for early disease diagnosis or prevention.